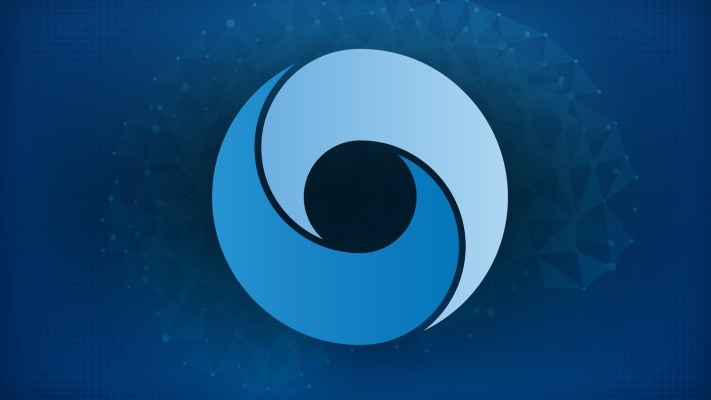
Almost 5 years in the past, DeepMind, considered one of Google’s extra prolific AI-centered analysis labs, debuted AlphaFold, an AI system that may precisely predict the buildings of many proteins contained in the human physique. Since then, DeepMind has improved on the system, releasing an up to date and extra succesful model of AlphaFold — AlphaFold 2 — in 2020.
And the lab’s work continues.
At the moment, DeepMind revealed that the latest launch of AlphaFold, the successor to AlphaFold 2, can generate predictions for practically all molecules within the Protein Information Financial institution, the world’s largest open entry database of organic molecules.
Already, Isomorphic Labs, a spin-off of DeepMind targeted on drug discovery, is making use of the brand new AlphaFold mannequin to therapeutic drug design, in keeping with a post on the DeepMind weblog — serving to to characterize various kinds of molecular buildings necessary for treating illness.
New capabilities
The brand new AlphaFold’s capabilities lengthen past protein prediction.
DeepMind claims that the mannequin also can precisely predict the buildings of ligands — molecules that bind to “receptor” proteins and trigger adjustments in how cells talk — in addition to nucleic acids (molecules that comprise key genetic data) and post-translational modifications (chemical adjustments that happen after a protein’s created).
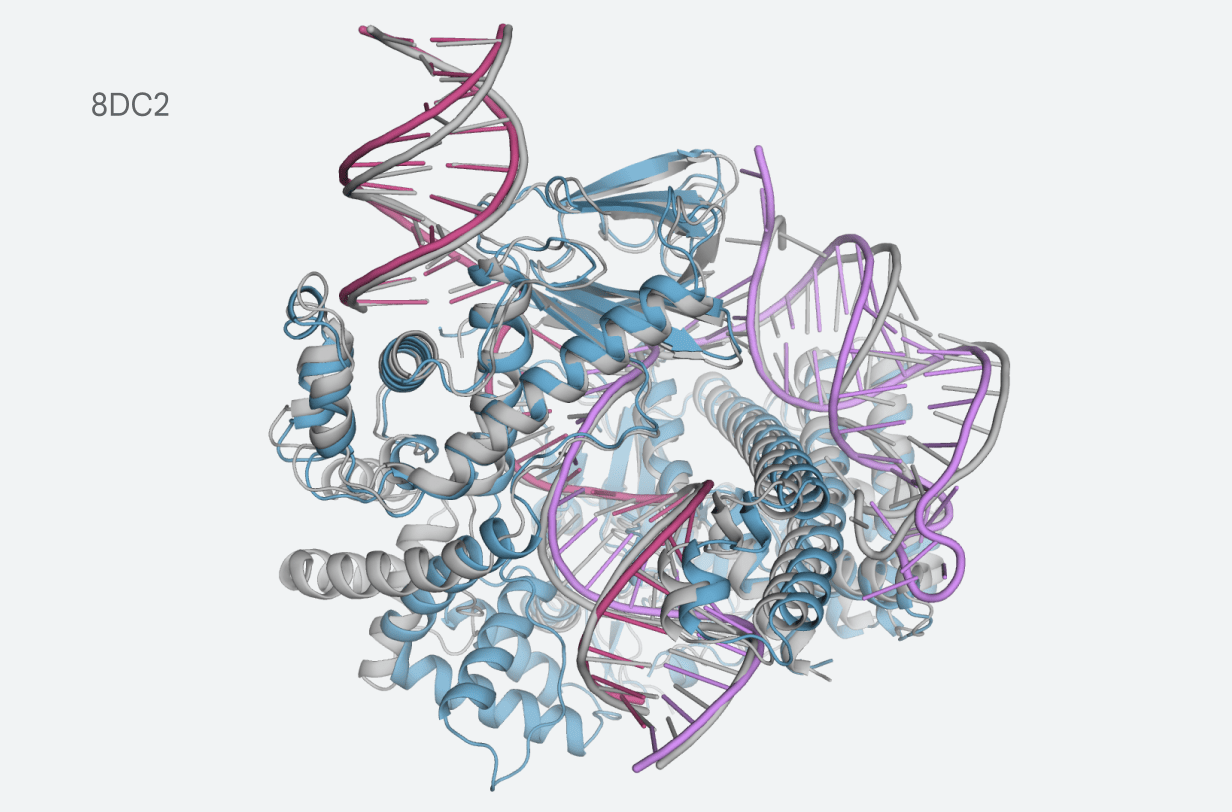
Picture Credit: DeepMind
Predicting protein-ligand buildings generally is a useful gizmo in drug discovery, DeepMind notes, as it will probably assist scientists establish and design new molecules that would turn into medicine.
At the moment, pharmaceutical researchers use pc simulations referred to as “docking strategies” to find out how proteins and ligands will work together. Docking strategies require specifying a reference protein construction and a prompt place on that construction for the ligand to bind to.
With the newest AlphaFold, nonetheless, there’s no want to make use of a reference protein construction or prompt place. The mannequin can predict proteins that haven’t been “structurally characterised” earlier than, whereas on the similar time simulating how proteins and nucleic acids work together with different molecules — a degree of modeling that DeepMind says isn’t potential with right now’s docking strategies.
“Early evaluation additionally exhibits that our mannequin drastically outperforms [the previous generation of] AlphaFold on some protein construction prediction issues which might be related for drug discovery, like antibody binding,” DeepMind writes within the put up. “Our mannequin’s dramatic leap in efficiency exhibits the potential of AI to drastically improve scientific understanding of the molecular machines that make up the human physique.”
The most recent AlphaFold isn’t good, although.
In a whitepaper detailing the system’s strengths and limitations, researchers at DeepMind and Isomorphic Labs reveal that the system falls in need of the best-in-class technique for predicting the buildings of RNA molecules — the molecules within the physique that carry the directions for making proteins.
Likely, each DeepMind and Isomorphic Labs are working to deal with this.