Reflecting on ChatGPT’s first 12 months, it is clear that this instrument has considerably modified the AI scene. Launched on the finish of 2022, ChatGPT stood out due to its user-friendly, conversational model that made interacting with AI really feel extra like chatting with an individual than a machine. This new method rapidly caught the general public’s eye. Inside simply 5 days after its launch, ChatGPT had already attracted 1,000,000 customers. By early 2023, this quantity ballooned to about 100 million month-to-month customers, and by October, the platform was drawing in round 1.7 billion visits worldwide. These numbers communicate volumes about its recognition and usefulness.
Over the previous 12 months, customers have discovered all types of artistic methods to make use of ChatGPT, from easy duties like writing emails and updating resumes to beginning profitable companies. However it’s not nearly how persons are utilizing it; the expertise itself has grown and improved. Initially, ChatGPT was a free service providing detailed textual content responses. Now, there’s ChatGPT Plus, which incorporates ChatGPT-4. This up to date model is educated on extra knowledge, offers fewer flawed solutions, and understands complicated directions higher.
One of many greatest updates is that ChatGPT can now work together in a number of methods – it will probably pay attention, communicate, and even course of photographs. This implies you may discuss to it by way of its cellular app and present it footage to get responses. These modifications have opened up new potentialities for AI and have modified how folks view and take into consideration AI’s function in our lives.
From its beginnings as a tech demo to its present standing as a significant participant within the tech world, ChatGPT’s journey is kind of spectacular. Initially, it was seen as a solution to take a look at and enhance expertise by getting suggestions from the general public. However it rapidly grew to become a necessary a part of the AI panorama. This success reveals how efficient it’s to fine-tune massive language fashions (LLMs) with each supervised studying and suggestions from people. Consequently, ChatGPT can deal with a variety of questions and duties.
The race to develop probably the most succesful and versatile AI programs has led to a proliferation of each open-source and proprietary fashions like ChatGPT. Understanding their common capabilities requires complete benchmarks throughout a large spectrum of duties. This part explores these benchmarks, shedding gentle on how totally different fashions, together with ChatGPT, stack up towards one another.
Evaluating LLMs: The Benchmarks
- MT-Bench: This benchmark exams multi-turn dialog and instruction-following talents throughout eight domains: writing, roleplay, data extraction, reasoning, math, coding, STEM data, and humanities/social sciences. Stronger LLMs like GPT-4 are used as evaluators.
- AlpacaEval: Primarily based on the AlpacaFarm analysis set, this LLM-based computerized evaluator benchmarks fashions towards responses from superior LLMs like GPT-4 and Claude, calculating the win fee of candidate fashions.
- Open LLM Leaderboard: Using the Language Mannequin Analysis Harness, this leaderboard evaluates LLMs on seven key benchmarks, together with reasoning challenges and common data exams, in each zero-shot and few-shot settings.
- BIG-bench: This collaborative benchmark covers over 200 novel language duties, spanning a various vary of subjects and languages. It goals to probe LLMs and predict their future capabilities.
- ChatEval: A multi-agent debate framework that enables groups to autonomously focus on and consider the standard of responses from totally different fashions on open-ended questions and conventional pure language era duties.
Comparative Efficiency
By way of common benchmarks, open-source LLMs have proven exceptional progress. Llama-2-70B, for example, achieved spectacular outcomes, notably after being fine-tuned with instruction knowledge. Its variant, Llama-2-chat-70B, excelled in AlpacaEval with a 92.66% win fee, surpassing GPT-3.5-turbo. Nevertheless, GPT-4 stays the frontrunner with a 95.28% win fee.
Zephyr-7B, a smaller mannequin, demonstrated capabilities akin to bigger 70B LLMs, particularly in AlpacaEval and MT-Bench. In the meantime, WizardLM-70B, fine-tuned with a various vary of instruction knowledge, scored the very best amongst open-source LLMs on MT-Bench. Nevertheless, it nonetheless lagged behind GPT-3.5-turbo and GPT-4.
An attention-grabbing entry, GodziLLa2-70B, achieved a aggressive rating on the Open LLM Leaderboard, showcasing the potential of experimental fashions combining numerous datasets. Equally, Yi-34B, developed from scratch, stood out with scores akin to GPT-3.5-turbo and solely barely behind GPT-4.
UltraLlama, with its fine-tuning on numerous and high-quality knowledge, matched GPT-3.5-turbo in its proposed benchmarks and even surpassed it in areas of world {and professional} data.
Scaling Up: The Rise of Large LLMs
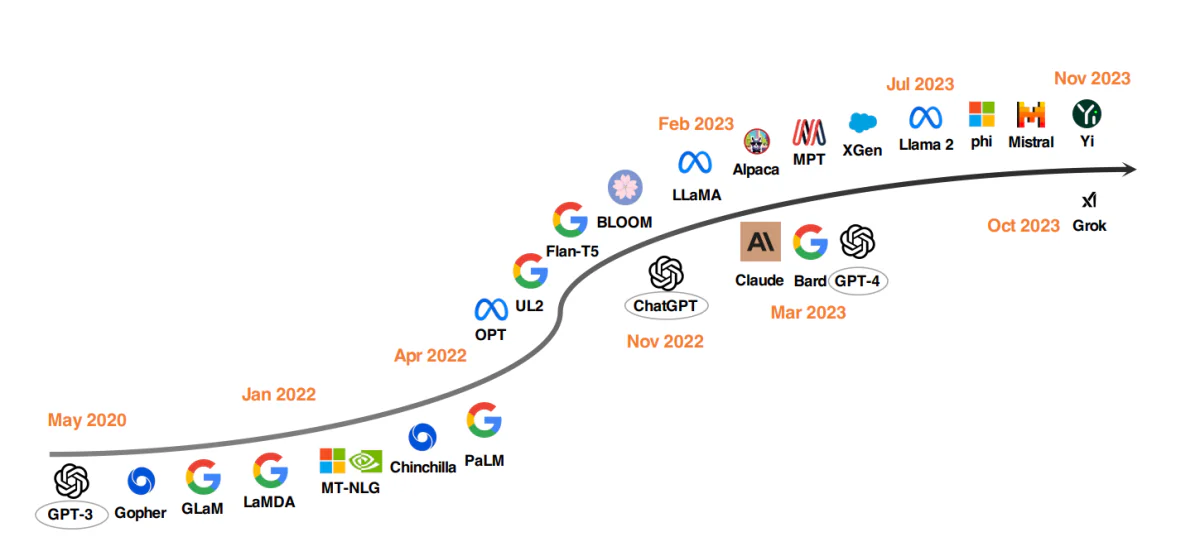
High LLM fashions since 2020
A notable development in LLM improvement has been the scaling up of mannequin parameters. Fashions like Gopher, GLaM, LaMDA, MT-NLG, and PaLM have pushed the boundaries, culminating in fashions with as much as 540 billion parameters. These fashions have proven distinctive capabilities, however their closed-source nature has restricted their wider utility. This limitation has spurred curiosity in growing open-source LLMs, a development that is gaining momentum.
In parallel to scaling up mannequin sizes, researchers have explored different methods. As an alternative of simply making fashions greater, they’ve targeted on bettering the pre-training of smaller fashions. Examples embrace Chinchilla and UL2, which have proven that extra is not all the time higher; smarter methods can yield environment friendly outcomes too. Moreover, there’s been appreciable consideration on instruction tuning of language fashions, with tasks like FLAN, T0, and Flan-T5 making important contributions to this space.
The ChatGPT Catalyst
The introduction of OpenAI’s ChatGPT marked a turning level in NLP analysis. To compete with OpenAI, firms like Google and Anthropic launched their very own fashions, Bard and Claude, respectively. Whereas these fashions present comparable efficiency to ChatGPT in lots of duties, they nonetheless lag behind the newest mannequin from OpenAI, GPT-4. The success of those fashions is primarily attributed to reinforcement studying from human suggestions (RLHF), a way that is receiving elevated analysis focus for additional enchancment.
Rumors and Speculations Round OpenAI’s Q* (Q-Star)
Recent reports counsel that researchers at OpenAI could have achieved a major development in AI with the event of a brand new mannequin known as Q* (pronounced Q star). Allegedly, Q* has the potential to carry out grade-school-level math, a feat that has sparked discussions amongst consultants about its potential as a milestone in the direction of synthetic common intelligence (AGI). Whereas OpenAI has not commented on these stories, the rumored talents of Q* have generated appreciable pleasure and hypothesis on social media and amongst AI fanatics.
The event of Q* is noteworthy as a result of current language fashions like ChatGPT and GPT-4, whereas able to some mathematical duties, will not be notably adept at dealing with them reliably. The problem lies within the want for AI fashions to not solely acknowledge patterns, as they presently do by way of deep studying and transformers, but in addition to motive and perceive summary ideas. Math, being a benchmark for reasoning, requires the AI to plan and execute a number of steps, demonstrating a deep grasp of summary ideas. This means would mark a major leap in AI capabilities, doubtlessly extending past arithmetic to different complicated duties.
Nevertheless, consultants warning towards overhyping this improvement. Whereas an AI system that reliably solves math issues can be a formidable achievement, it does not essentially sign the arrival of superintelligent AI or AGI. Present AI analysis, together with efforts by OpenAI, has targeted on elementary issues, with various levels of success in additional complicated duties.
The potential functions developments like Q* are huge, starting from personalised tutoring to helping in scientific analysis and engineering. Nevertheless, it is also necessary to handle expectations and acknowledge the restrictions and security considerations related to such developments. The considerations about AI posing existential dangers, a foundational fear of OpenAI, stay pertinent, particularly as AI programs start to interface extra with the actual world.
The Open-Supply LLM Motion
To spice up open-source LLM analysis, Meta launched the Llama collection fashions, triggering a wave of latest developments primarily based on Llama. This contains fashions fine-tuned with instruction knowledge, reminiscent of Alpaca, Vicuna, Lima, and WizardLM. Analysis can be branching into enhancing agent capabilities, logical reasoning, and long-context modeling throughout the Llama-based framework.
Moreover, there is a rising development of growing highly effective LLMs from scratch, with tasks like MPT, Falcon, XGen, Phi, Baichuan, Mistral, Grok, and Yi. These efforts mirror a dedication to democratize the capabilities of closed-source LLMs, making superior AI instruments extra accessible and environment friendly.
The Influence of ChatGPT and Open Supply Fashions in Healthcare
We’re a future the place LLMs help in scientific note-taking, form-filling for reimbursements, and supporting physicians in prognosis and therapy planning. This has caught the eye of each tech giants and healthcare establishments.
Microsoft’s discussions with Epic, a number one digital well being data software program supplier, sign the mixing of LLMs into healthcare. Initiatives are already in place at UC San Diego Well being and Stanford College Medical Middle. Equally, Google’s partnerships with Mayo Clinic and Amazon Web Services‘ launch of HealthScribe, an AI scientific documentation service, mark important strides on this course.
Nevertheless, these speedy deployments increase considerations about ceding management of drugs to company pursuits. The proprietary nature of those LLMs makes them tough to guage. Their attainable modification or discontinuation for profitability causes might compromise affected person care, privateness, and security.
The pressing want is for an open and inclusive method to LLM improvement in healthcare. Healthcare establishments, researchers, clinicians, and sufferers should collaborate globally to construct open-source LLMs for healthcare. This method, just like the Trillion Parameter Consortium, would permit pooling of computational, monetary sources, and experience.