Current progress in AI largely boils down to at least one factor: Scale.
Across the starting of this decade, AI labs seen that making their algorithms—or fashions—ever larger and feeding them extra information persistently led to huge enhancements in what they may do and the way nicely they did it. The newest crop of AI fashions have tons of of billions to over a trillion inner community connections and be taught to write down or code like we do by consuming a wholesome fraction of the web.
It takes extra computing energy to coach larger algorithms. So, to get thus far, the computing devoted to AI coaching has been quadrupling yearly, based on nonprofit AI analysis group, Epoch AI.
Ought to that development proceed via 2030, future AI fashions could be skilled with 10,000 instances extra compute than right now’s cutting-edge algorithms, like OpenAI’s GPT-4.
“If pursued, we would see by the tip of the last decade advances in AI as drastic because the distinction between the rudimentary textual content era of GPT-2 in 2019 and the delicate problem-solving talents of GPT-4 in 2023,” Epoch wrote in a recent research report detailing how doubtless it’s this situation is feasible.
However fashionable AI already sucks in a major quantity of energy, tens of 1000’s of superior chips, and trillions of on-line examples. In the meantime, the business has endured chip shortages, and research recommend it might run out of high quality coaching information. Assuming corporations proceed to spend money on AI scaling: Is development at this charge even technically potential?
In its report, Epoch checked out 4 of the most important constraints to AI scaling: Energy, chips, information, and latency. TLDR: Sustaining development is technically potential, however not sure. Right here’s why.
Energy: We’ll Want a Lot
Energy is the most important constraint to AI scaling. Warehouses filled with superior chips and the gear to make them run—or information facilities—are energy hogs. Meta’s newest frontier mannequin was skilled on 16,000 of Nvidia’s strongest chips drawing 27 megawatts of electrical energy.
This, based on Epoch, is the same as the annual energy consumption of 23,000 US households. However even with effectivity beneficial properties, coaching a frontier AI mannequin in 2030 would wish 200 instances extra energy, or roughly 6 gigawatts. That’s 30 p.c of the facility consumed by all information facilities right now.
There are few energy crops that may muster that a lot, and most are doubtless below long-term contract. However that’s assuming one energy station would electrify an information middle. Epoch suggests corporations will search out areas the place they will draw from a number of energy crops by way of the native grid. Accounting for deliberate utilities development, going this route is tight however potential.
To higher break the bottleneck, corporations could as a substitute distribute coaching between a number of information facilities. Right here, they’d break up batches of coaching information between various geographically separate information facilities, lessening the facility necessities of anybody. The technique would require lightning-quick, high-bandwidth fiber connections. But it surely’s technically doable, and Google Gemini Extremely’s coaching run is an early instance.
All advised, Epoch suggests a spread of potentialities from 1 gigawatt (native energy sources) all the way in which as much as 45 gigawatts (distributed energy sources). The extra energy corporations faucet, the bigger the fashions they will practice. Given energy constraints, a mannequin could possibly be skilled utilizing about 10,000 instances extra computing energy than GPT-4.
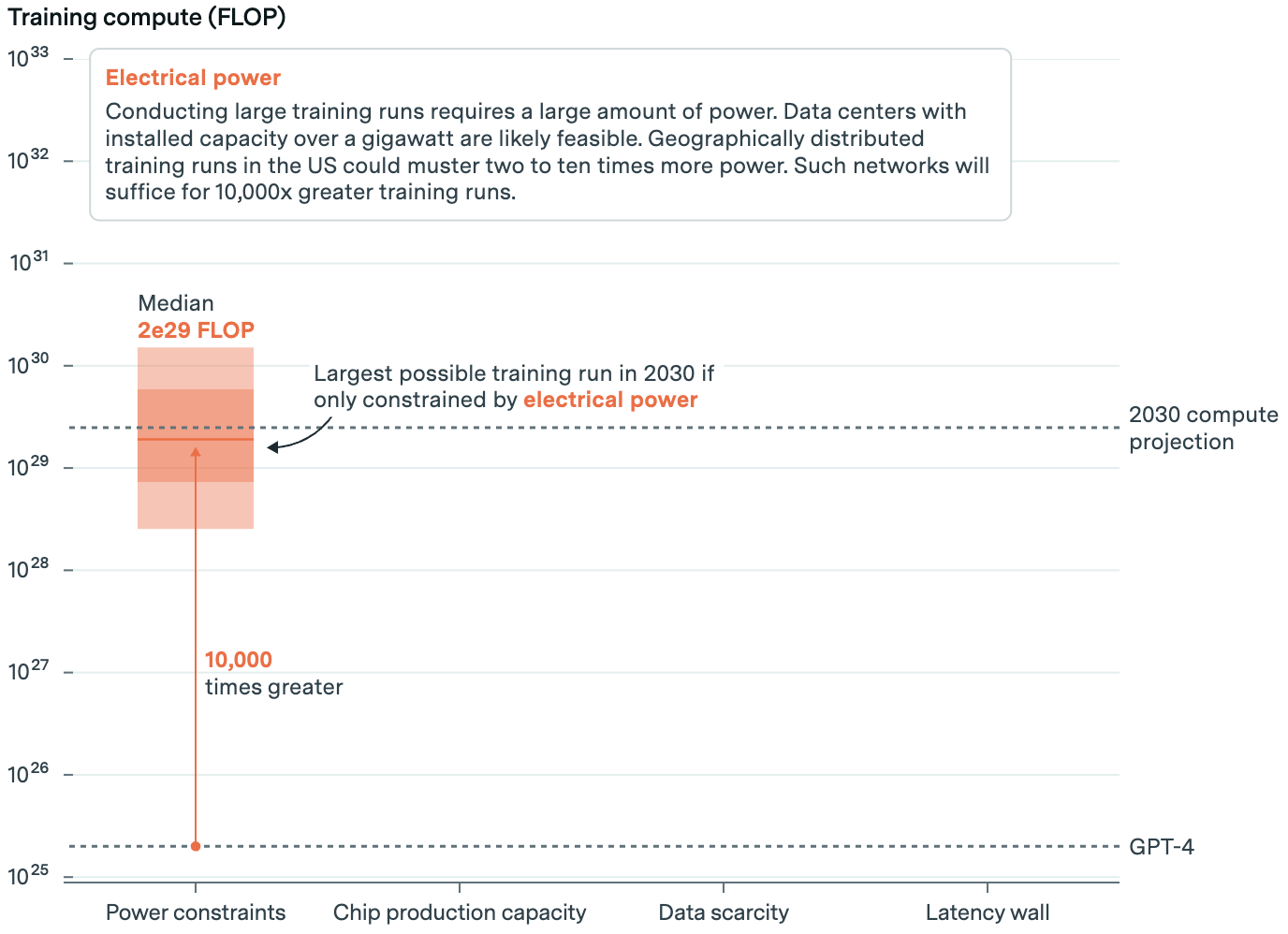
Chips: Does It Compute?
All that energy is used to run AI chips. A few of these serve up accomplished AI fashions to clients; some practice the following crop of fashions. Epoch took a detailed have a look at the latter.
AI labs practice new fashions utilizing graphics processing models, or GPUs, and Nvidia is high canine in GPUs. TSMC manufactures these chips and sandwiches them along with high-bandwidth reminiscence. Forecasting has to take all three steps under consideration. In accordance with Epoch, there’s doubtless spare capability in GPU manufacturing, however reminiscence and packaging could maintain issues again.
Given projected business development in manufacturing capability, they assume between 20 and 400 million AI chips could also be accessible for AI coaching in 2030. A few of these will likely be serving up current fashions, and AI labs will solely be capable to purchase a fraction of the entire.
The big selection is indicative of quantity of uncertainty within the mannequin. However given anticipated chip capability, they consider a mannequin could possibly be skilled on some 50,000 instances extra computing energy than GPT-4.
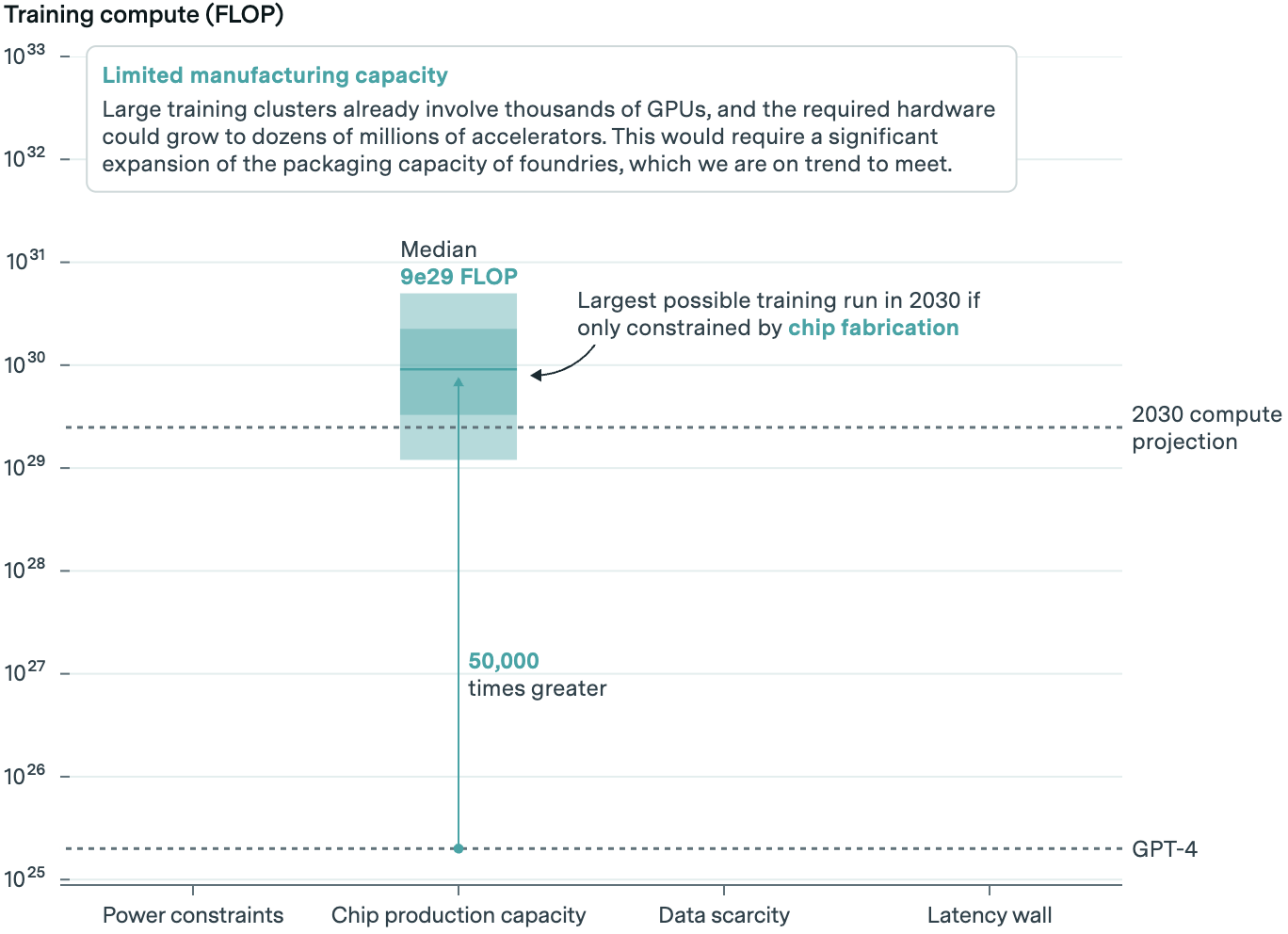
Knowledge: AI’s On-line Schooling
AI’s starvation for information and its impending shortage is a well known constraint. Some forecast the stream of high-quality, publicly accessible information will run out by 2026. However Epoch doesn’t assume information shortage will curtail the expansion of fashions via no less than 2030.
At right now’s development charge, they write, AI labs will run out of high quality textual content information in 5 years. Copyright lawsuits might also affect provide. Epoch believes this provides uncertainty to their mannequin. However even when courts resolve in favor of copyright holders, complexity in enforcement and licensing offers like these pursued by Vox Media, Time, The Atlantic and others imply the affect on provide will likely be restricted (although the standard of sources could endure).
However crucially, fashions now eat extra than simply textual content in coaching. Google’s Gemini was skilled on picture, audio, and video information, for instance.
Non-text information can add to the availability of textual content information by means of captions and transcripts. It may possibly additionally broaden a mannequin’s talents, like recognizing the meals in a picture of your fridge and suggesting dinner. It could even, extra speculatively, lead to switch studying, the place fashions skilled on a number of information sorts outperform these skilled on only one.
There’s additionally proof, Epoch says, that artificial information might additional develop the information haul, although by how a lot is unclear. DeepMind has lengthy used artificial information in its reinforcement studying algorithms, and Meta employed some artificial information to coach its newest AI fashions. However there could also be arduous limits to how a lot can be utilized with out degrading mannequin high quality. And it might additionally take much more—expensive—computing energy to generate.
All advised, although, together with textual content, non-text, and artificial information, Epoch estimates there’ll be sufficient to coach AI fashions with 80,000 instances extra computing energy than GPT-4.
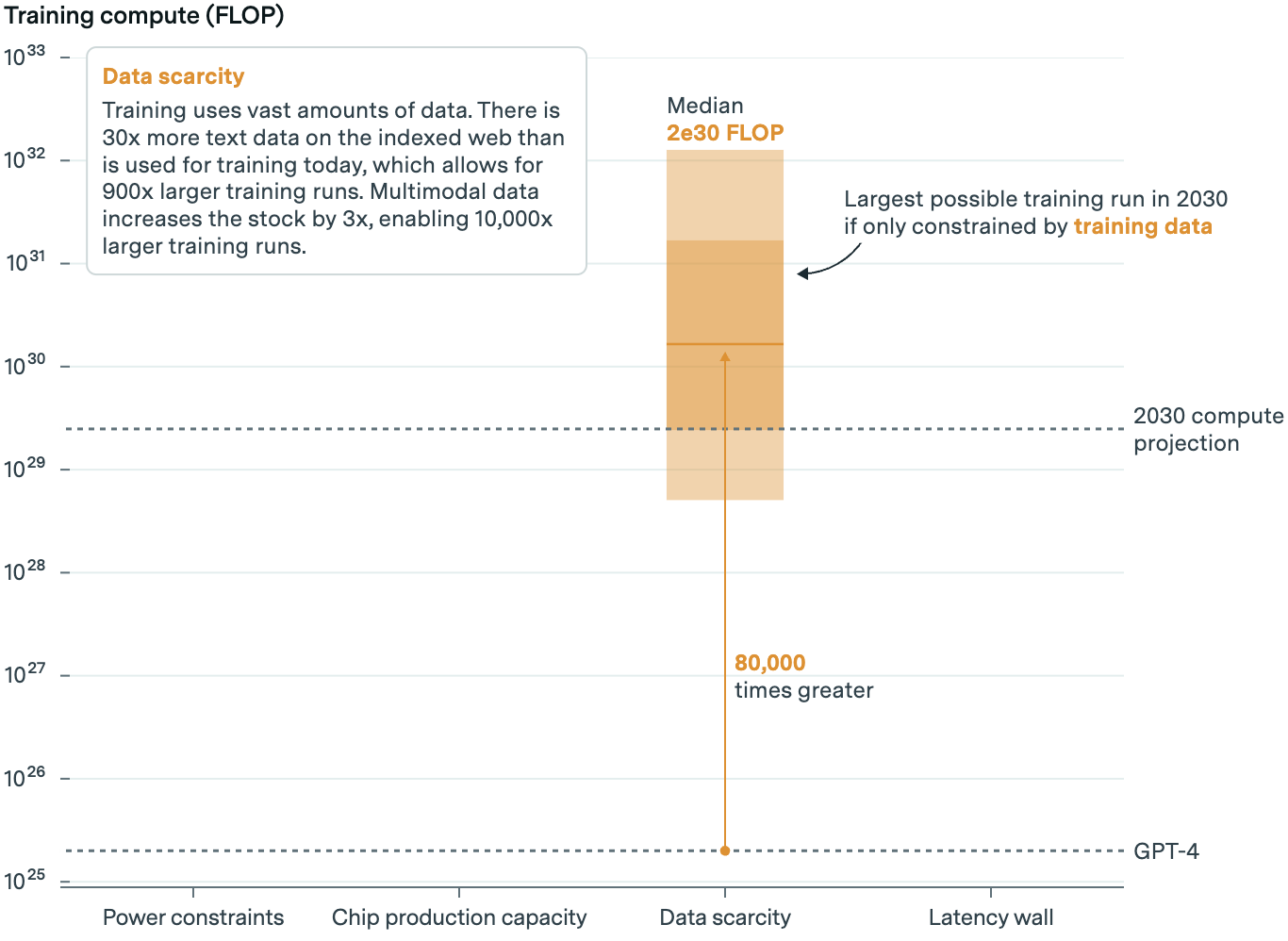
Latency: Larger Is Slower
The final constraint is expounded to the sheer measurement of upcoming algorithms. The larger the algorithm, the longer it takes for information to traverse its community of synthetic neurons. This might imply the time it takes to coach new algorithms turns into impractical.
This bit will get technical. Briefly, Epoch takes a have a look at the potential measurement of future fashions, the dimensions of the batches of coaching information processed in parallel, and the time it takes for that information to be processed inside and between servers in an AI information middle. This yields an estimate of how lengthy it might take to coach a mannequin of a sure measurement.
The primary takeaway: Coaching AI fashions with right now’s setup will hit a ceiling finally—however not for awhile. Epoch estimates that, below present practices, we might practice AI fashions with upwards of 1,000,000 instances extra computing energy than GPT-4.
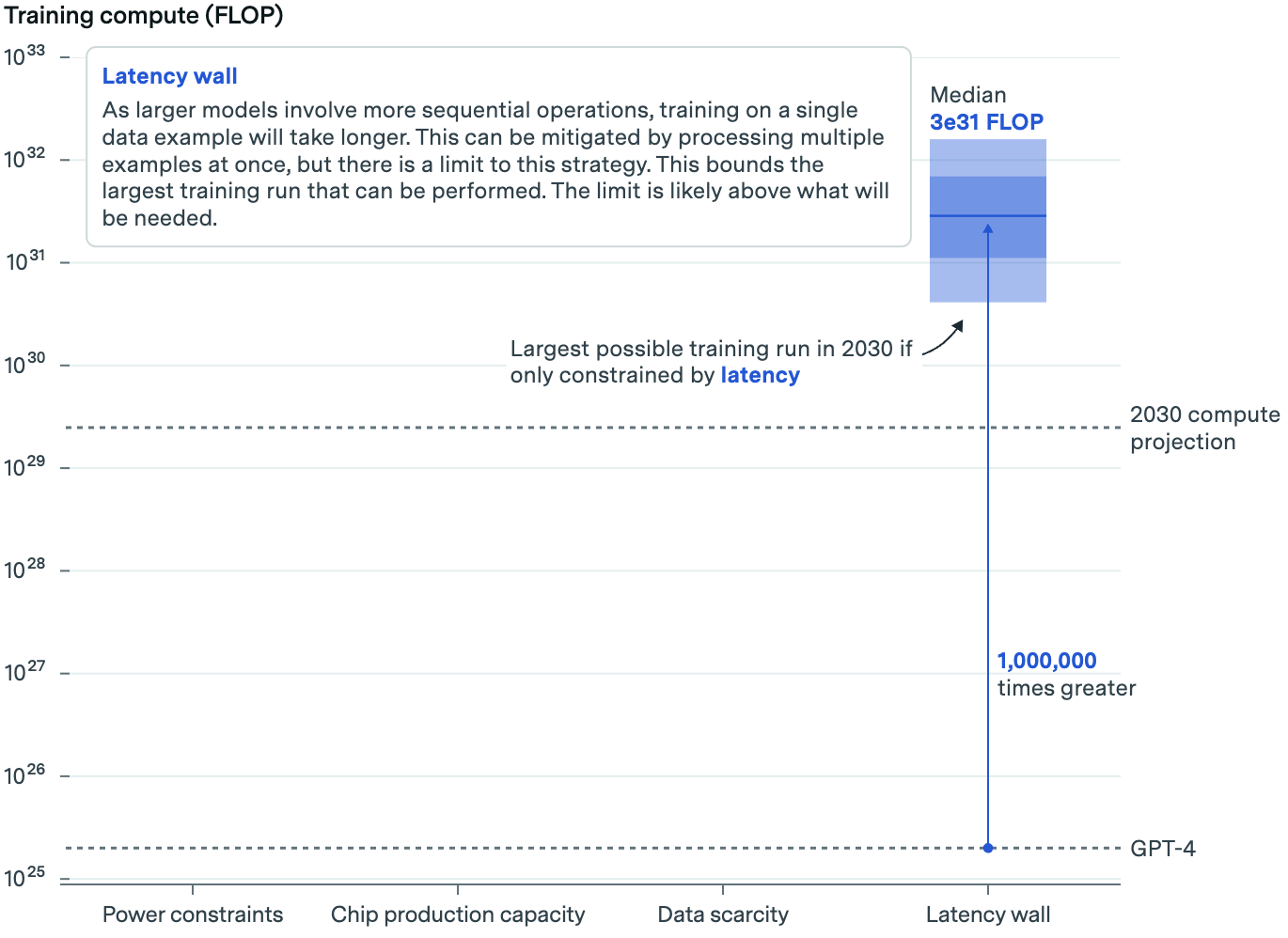
Scaling Up 10,000x
You’ll have seen the size of potential AI fashions will get bigger below every constraint—that’s, the ceiling is greater for chips than energy, for information than chips, and so forth. But when we contemplate all of them collectively, fashions will solely be potential as much as the primary bottleneck encountered—and on this case, that’s energy. Even so, important scaling is technically potential.
“When thought of collectively, [these AI bottlenecks] suggest that coaching runs of as much as 2e29 FLOP could be possible by the tip of the last decade,” Epoch writes.
“This is able to symbolize a roughly 10,000-fold scale-up relative to present fashions, and it might imply that the historic development of scaling might proceed uninterrupted till 2030.”
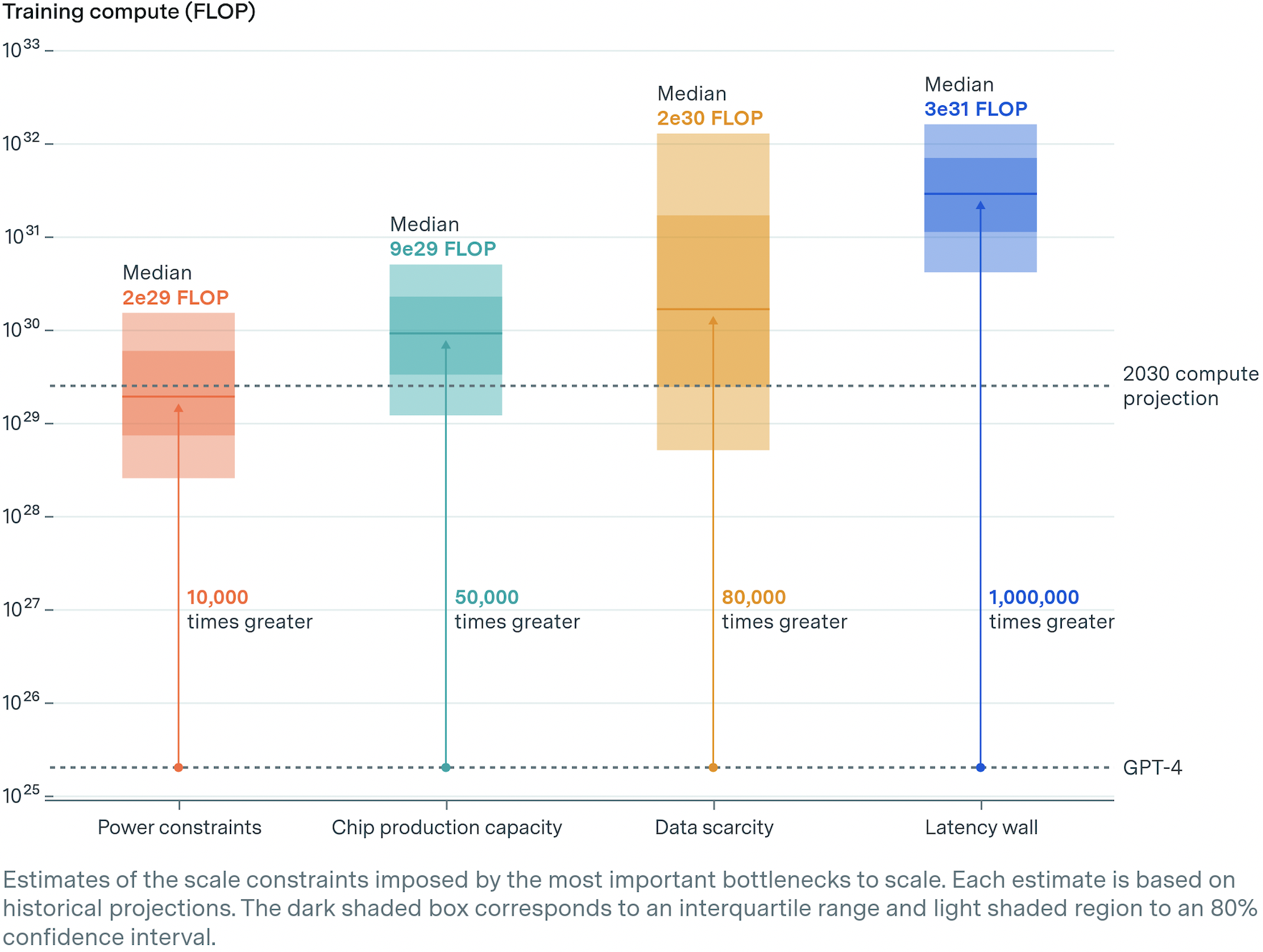
What Have You Achieved for Me Currently?
Whereas all this means continued scaling is technically potential, it additionally makes a fundamental assumption: That AI funding will develop as wanted to fund scaling and that scaling will proceed to yield spectacular—and extra importantly, helpful—advances.
For now, there’s each indication tech corporations will hold investing historic quantities of money. Pushed by AI, spending on the likes of latest tools and actual property has already jumped to levels not seen in years.
“If you undergo a curve like this, the danger of underinvesting is dramatically larger than the danger of overinvesting,” Alphabet CEO Sundar Pichai mentioned on final quarter’s earnings name as justification.
However spending might want to develop much more. Anthropic CEO Dario Amodei estimates fashions skilled right now can value as much as $1 billion, subsequent 12 months’s fashions could close to $10 billion, and prices per mannequin might hit $100 billion within the years thereafter. That’s a dizzying quantity, but it surely’s a price ticket corporations could also be keen to pay. Microsoft is already reportedly committing that a lot to its Stargate AI supercomputer, a joint venture with OpenAI due out in 2028.
It goes with out saying that the urge for food to speculate tens or tons of of billions of {dollars}—greater than the GDP of many nations and a major fraction of present annual revenues of tech’s largest gamers—isn’t assured. Because the shine wears off, whether or not AI development is sustained could come right down to a query of, “What have you ever accomplished for me these days?”
Already, buyers are checking the underside line. At the moment, the amount invested dwarfs the amount returned. To justify larger spending, companies should present proof that scaling continues to provide increasingly more succesful AI fashions. Which means there’s rising strain on upcoming fashions to transcend incremental enhancements. If beneficial properties tail off or sufficient individuals aren’t keen to pay for AI merchandise, the story could change.
Additionally, some critics consider massive language and multimodal fashions will show to be a pricy dead end. And there’s at all times the prospect a breakthrough, just like the one which kicked off this spherical, reveals we will accomplish extra with much less. Our brains be taught repeatedly on a light-weight bulb’s value of vitality and nowhere close to an web’s value of information.
That mentioned, if the present strategy “can automate a considerable portion of financial duties,” the monetary return might quantity within the trillions of {dollars}, greater than justifying the spend, based on Epoch. Many within the business are keen to take that wager. Nobody is aware of the way it’ll shake out but.
Picture Credit score: Werclive 👹 / Unsplash