Rick Korzekwa, February 1, 2023
Word: This publish is meant to be accessible to readers with comparatively little background in AI security. Folks with a agency understanding of AI security could discover it too primary, although they might be eager about figuring out which sorts of insurance policies I’ve been encountering or telling me if I’ve gotten one thing flawed.
I’ve lately encountered many proposals for insurance policies and rules supposed to scale back threat from superior AI. These proposals are extremely diversified and most of them appear to be well-thought-out, no less than on some axes. However lots of them fail to confront the technical and strategic realities of safely creating highly effective AI, they usually usually fail in related methods. On this publish, I’ll describe a standard kind of proposal and provides a primary overview of the explanation why it’s insufficient. I can’t handle any points associated to the feasibility of implementing such a coverage.
Caveats
- It’s seemingly that I’ve misunderstood some proposals and that they’re already addressing my issues. Furthermore, I don’t suggest dismissing a proposal as a result of it pattern-matches to product security on the floor stage.
- These approaches could also be good when mixed with others. It’s believable to me that an efficient and complete method to governing AI will embody product-safety-like rules, particularly early within the course of after we’re nonetheless studying and setting the groundwork for extra mature insurance policies.
The product security mannequin of AI governance
A typical AI coverage construction, which I’ll name the ‘product security mannequin of AI governance’, appears to be constructed on the belief that, whereas the processes concerned in creating highly effective AI could should be regulated, the hurt from a failure to make sure security happens predominantly when the AI has been deployed into the world. Beneath this mannequin, the first suggestions loop for guaranteeing security relies on the habits of the mannequin after it has been constructed. The product is developed, evaluated for security, and both despatched again for extra improvement or allowed to be deployed, relying on the analysis. For a typical instance, here’s a diagram from a US Division of Protection report on accountable AI:
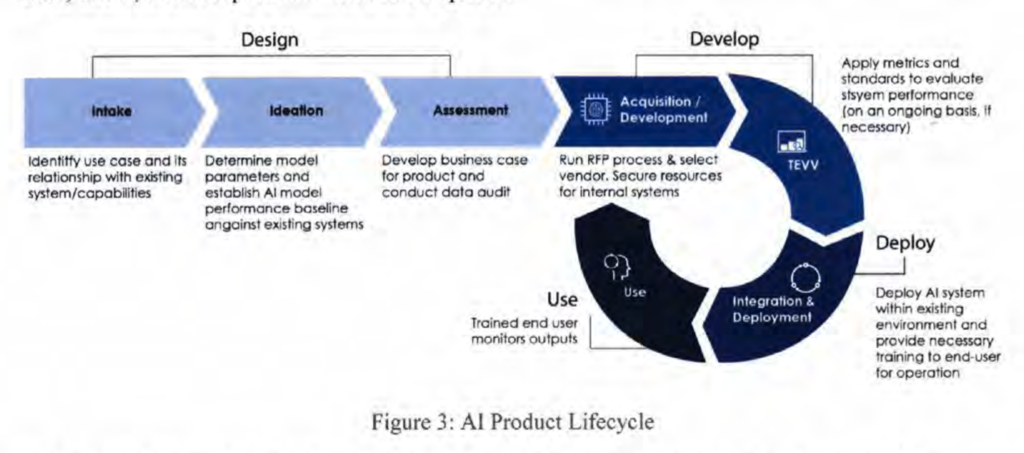
The system on this diagram will not be formally evaluated for security or efficiency till after “Acquisition/Growth”.
I don’t discover it shocking that this mannequin is so frequent. More often than not after we are involved about threat from know-how, we’re fearful about what occurs when the know-how has been launched into the world. A defective brake line on a automobile will not be a lot of a priority to the general public till the automobile is on public roads, and the fb feed algorithm can’t be a menace to society till it’s used to regulate what massive numbers of individuals see on their screens. I additionally suppose it’s affordable to start out with rules which can be already well-understood, and work from there. However this mannequin, by itself, is insufficient for AI, for causes I’ll clarify within the subsequent part.
This mannequin fails when utilized to superior AI
This method can’t be relied on to forestall the most important dangers related to superior AI. I’ll give two causes for this, although there could also be others.
Security can not (but) be evaluated reliably in a fully-developed AI
A elementary problem of guaranteeing that AI will behave as supposed is that inspecting an AI or observing its habits throughout improvement and testing will not be a dependable indicator of the way it will behave as soon as deployed. It is a troublesome, unsolved downside that resists easy options. I can’t clarify this downside intimately, however I’ll listing some features of it right here, together with real-world examples, and you may learn extra about it elsewhere.
AI could study approaches to issues or, if sufficiently superior, purchase objectives that end in habits that appears to work in coaching however will fail in deployment.
- A system educated to categorise photos carried out extraordinarily nicely, however solely as a result of it had discovered to detect photos based mostly on their storage location.
- A simulated robotic was educated utilizing human suggestions. It was supposed to understand an object, however as an alternative it discovered to put its hand between the thing and the digicam, giving the looks of greedy it.
AI can discover options to issues that can’t be reliably evaluated by people.
- An evolutionary algorithm for designing circuits on an actual circuit board created a circuit that produced the specified output, however the researchers had been unable to find out the way it labored.
AI with objectives which can be at odds with human pursuits can have an incentive to keep away from habits that reveals its objectives.
- Creatures present process simulated evolution discovered to determine once they had been in an analysis atmosphere and once they had been within the “actual” atmosphere, and used this to cover their undesirable habits.
Unsafe AI could cause substantial hurt earlier than deployment
Not like most applied sciences, AI can pose a severe threat even throughout improvement and testing. As AI capabilities method and surpass human capabilities, the capability for AI to trigger hurt will enhance and it’ll grow to be tougher to make sure that AI is unable to trigger hurt outdoors its improvement and testing atmosphere. I can’t go into a lot of element on this, however I’ll define the essential causes for concern.
AI is prone to search affect outdoors its supposed area
Whether or not a given AI system will try to realize affect outdoors the atmosphere by which it’s educated and examined is troublesome to find out, however now we have ample purpose to be cautious. Studying to create and execute plans towards a specific purpose is one thing we must always anticipate by default inside a broad vary of AI functions as capabilities enhance, and for a system to do nicely on real-world duties, it might want to make use of details about the broader world. That is one thing that present language fashions are already doing on their very own. There are additionally examples of AI discovering vulnerabilities that permit it to control issues it was not supposed to have entry to, comparable to a game-playing AI that exploited a glitch within the recreation to extend its rating instantly.
It’s troublesome to constrain superior AI to a specific area
AI with entry to the Web could create copies of itself, acquire entry to influential methods comparable to essential infrastructure, or manipulate individuals into taking actions which can be dangerous or enhance its affect. AI with out direct entry to the skin world might be able to acquire entry by manipulating individuals concerned in its improvement or by exploiting {hardware} vulnerabilities.
Executing such an escape from a datacenter could not require very excessive functionality. Surprisingly easy social engineering strategies could be profitable at getting an individual to do one thing that isn’t of their curiosity. Malware that may propagate between computer systems and management them has existed for a very long time and doesn’t require the software program to have any high-level choice making functionality in any respect.
Importantly, detecting such escape makes an attempt could also be very troublesome. Virtually any AI can have a powerful incentive to not be noticed taking harmful, unintended actions, and present methods generally discover unintended options which can be invisible to people. Moreover, AI in coaching is topic to the analysis difficulties defined within the first part.
AI breakout may very well be catastrophic
As soon as AI has gained affect outdoors its supposed area, it may trigger immense hurt. How seemingly that is to occur and the way a lot hurt it might trigger is a giant matter that I can’t attempt to cowl right here, however there are some things value stating on this context:
- Many of the arguments round catastrophic AI threat are agnostic as to if AI positive aspects affect earlier than or after deployment.
- The essential premise behind catastrophic AI threat isn’t just that it might use no matter affect it’s granted to trigger hurt, however that it’ll search extra affect. That is at odds with the essential concept behind the product security mannequin, which is that we forestall hurt by solely granting affect to AI that has been verified as secure.
- The general stakes are a lot larger than these related to issues we usually apply the product security mannequin to, and in contrast to most dangers from know-how, the prices are huge reaching and nearly totally exterior.
Extra feedback
I’m reluctant to supply various fashions, partly as a result of I wish to preserve the scope of this publish slender, but additionally as a result of I’m unsure which approaches are viable. However I can no less than present some guiding rules:
- Insurance policies supposed to mitigate threat from superior AI should acknowledge that verifying the protection of extremely succesful AI methods is an unsolved downside which will stay unsolved for the foreseeable future.
- These insurance policies should additionally acknowledge that public threat from AI begins throughout improvement, not on the time of deployment.
- Extra broadly, you will need to view AI threat as distinct from most different applied sciences. It’s poorly understood, troublesome to regulate in a dependable and verifiable method, and should grow to be very harmful earlier than it turns into nicely understood.
Lastly, I feel you will need to emphasize that, whereas it’s tempting to attend till we see the warning indicators that AI is turning into harmful earlier than enacting such insurance policies, capabilities are advancing quickly and AI could purchase the flexibility to hunt energy and conceal its actions in a classy method with comparatively little warning.
Acknowledgements
Due to Harlan Stewart for giving suggestions and serving to with citations, Irina Gueorguiev for productive conversations on this matter, and Aysja Johnson, Jeffrey Heninger, and Zach Stein-Perlman for suggestions on an earlier draft. All errors are my very own.